
Data-Driven Discoveries in a Changing Climate KB-46)
With Wageningen climate solutions, we at WUR are jointly developing opportunities to improve local quality of life. The fact that WUR is a world-leading research organisation is because we successfully integrate fundamental and applied research from different perspectives. This interdisciplinary approach to problems is known as the 'Wageningen approach'.
Exploring the opportunities offered by AI for data-driven discovery to better understand climate change impacts and develop new solutions to adapt to a changing climate.
Towards climate smart systems
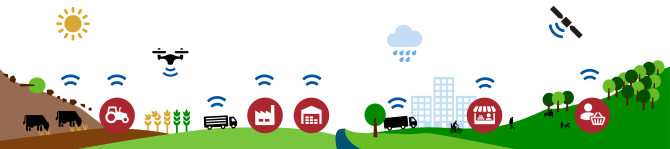
Investment theme D3-C2 aims to advance Wageningen Climate Solutions by exploring the potential of AI for data-driven discovery. In this way, we aim to better understand the effects of climate change and develop new solutions to adapt to a changing climate.
Together, create climate smart systems and map them to nature-based solutions. In doing so, we increase climate resilience and work together across disciplines. In addition, bringing together the vast amounts of data available at WUR, and from our partners, is of great importance. In doing so, we are especially looking at the effects of climate change on different socio-ecological systems.
Download the posters
Climate impacts
2022
New data-driven solutions
- Impact of climate change on dairy cow welfare using high-frequent behavioural sensor data
- AI-driven discovery of the linkage between climate extremes and food fraud
Emerging DS/AI methods
Climate adaptation solutions
2022
New data-driven solutions
- Heat stress detection in broilers using sensor data
- I-KNOW-FOO: Interlinking and creating KNOWledgegraphs for near-zero CO2 emission diets and climate-change robust FOOd production
- AI techniques to evaluate the effectiveness of nature-based solutions in reducing urban heat islands
- Decision support system for adapting sowing dates for maximum productivity
- ML-CLIMATE
- Enhancing drought resilience through forest restoration
Emerging DS/AI methods
- Integrating machine learning and mechanistic modelling towards adaptation of plants to climate change
- Use AI to Improve Climate Change Adaptation of Smart Greenhouse
Open data challenges
Download the factsheets
2023
- AI driven discovery of the linkage between climate extremes and food fraud
- Discover the impact of climate change on dairy cow welfare using high-frequent behavioural sensor data
- A digital twin of the global water and food system
- BreedGym
- Scientific machine learning to simplify hydrological modelling in a changing climate
- Use AI to improve climate change adaptation of smart greenhouse
- Climate impact on agricultural labour productivity
- AI techniques to evaluate the effectiveness of nature-based solutions in reducing urban heat islands
- ML-CLIMATE
- Turing meets Turing
- Integration of machine learning and mechanistic modelling towards adaptation of plants to climate change
- Enhancing drought resilience in Africa through large-scale tree planting
- Heat stress reduction in broilers using sensor data
2024
- Assessment of realistic climate change impacts of cropping seasons
- Machine learning for selecting crop varieties as climate adaptation measure
- Yield loss information from machine learning for insurance payouts
- Deep-learning-based streamflow monitor
- Climate impact at dairy farms
- Machine learning to identify good practices with Nature-based Solution
- AI4Chocolate
- ASPAR_KR
- Predicting drought and heatwave effects
Action plan
How are we going to do it?
Mission and vision
Our vision is that ai methodology will enable us to identify patterns in data to better understand the impacts of climate change, develop new solutions for adapting to a changing climate and prepare for climate action. Data-driven discovery can be the key element in accelerating the identification, development and acceptance of evidence-based solutions for adaptation to a changing climate.
Design climate change adaptation solutions, including both nature-based solutions and climate smart systems. Nature-based solutions, such as forest and landscape restoration, have also been widely recognised to enhance climate resilience. However, empirical evidence in this regard is scarce. For example, quantifying where and to what extent vegetation restoration can improve drought resilience in vulnerable areas in a changing climate remains a challenge. In this line of research, we will explore opportunities to develop nature-based futures by developing scenarios from data. For example, generative machine learning approaches for generating scenarios. For this, advanced visualisation techniques (c.f. WANDER) can be used synergistically.
Challenges
Three sub-themes have been identified where adaptation to climate change in food systems and the living environment can benefit from data-driven discoveries:
- to improve our understanding of the problem by analysing and visualising historical or simulated data on climate change impacts;
- to design effective solutions for adaptation to a changing climate, including both nature-based solutions and climate-smart systems;
- to monitor the effectiveness of climate adaptation policies and public engagement.
Ambition
Our desired outcomes for research are:
- New data-driven solutions for climate change adaptation - Projects will help monitor and analyse climate impact data to develop evidence-based solutions. Innovations may lie in the identification or assessment of climate change adaptation options by implementing DS/AI techniques already used in other areas/domains. This is where the innovativeness comes from interdisciplinary research. This will affect climate change adaptation measures in the short term.
- Broaden and strengthen the Portfolio Van Wageningen Climate Solutions - Projects are underway to design climate adaptation solutions for climate smart systems, both nature-based solutions and climate smart systems. Innovations will come from methodological breakthroughs, developing new AI techniques for the specific problems and research questions of climate change adaptation. This will have long-term implications for climate change adaptation measures.
- Strengthen WUR's DS/AI expertise - Successfully applying new or existing DS/AI methods to specific climate change challenges requires the development of new expertise within WUR to deliver fruitful results: bringing together existing data with computational experiments and the extensive involvement of domain expertise.
- Improve WUR's visibility in the DS/AI community - This will attract DS/AI from outside WUR to connect with our domain expertise. This is where we connect to challenges with long-term impact for WUR. This will also strengthen our partnerships with networks such as the Dutch AI Coalition, ICAI labs and Climate Change AI, and major technology companies, such as IBM, Google, Microsoft and data platforms.
- Improve visibility wageningen climate solutions - New data-driven discoveries strengthen WUR's position in the global climate debate. WUR will strengthen its position as a leading global centre for climate change adaptation research by integrating DS/AI, which are essentially multiplicative and bring new collaborations.
Deliverables / research lines
- The investment theme D3-C2 aims to support collaborative grants, which clearly address one of the three thematic challenges (Figure 2), and have the ambition to;
- Advance emerging DS/AI methods by exploring new methods and developing AI systems that contribute to climate change adaptation research. These projects should show how they strengthen WUR's DS/AI expertise portfolio and have high academic ambition; or
- develop new data-driven climate change adaptation solutions, by bringing together established data science methods and WUR domain expertise to jointly develop new, ambitious climate research solutions. Projects in this category aim to broaden the WUR climate research portfolio, with the ambition to develop new impactful applications that address long-term climate change adaptation problems; or
- organising open data challenges for climate adaptation, by bringing together big climate data from WUR and global open data facilities (e.g. Copernicus and GBIF) and formulating relevant DS/AI problems. In this way, we can attract (external) AI talent to engage with WUR and contribute to climate change adaptation research. Domain expertise from WUR will be a catalyst for defining appropriate climate problems to which the DS/AI community will be invited to contribute. Projects in this category will clearly target specific DS/AI conferences to present their developed data challenge along with statistics and benchmark data.
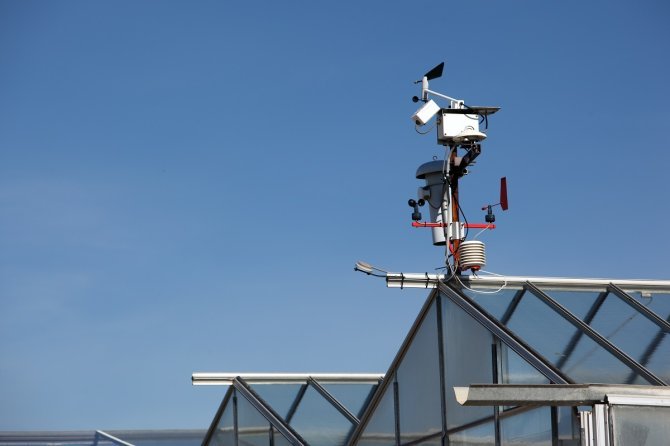
An integrated approach
Most initiatives originate from the AI community and develop separately from domain knowledge. This hinders the full potential for data-driven discovery. WUR is working on an integrated approach:domain knowledge deploys AI expertise.
This is needed to realise major breakthroughs. Moreover, while AI has been extensively researched for climate mitigation solutions, there is less experience on how DS and AI methods can contribute to the development of new climate adaptation solutions.
Wageningen University & Research is uniquely positioned internationally and nationally, to fill this research niche. Recent investments in DS/AI provide WUR with the basis to build a strong profile that combines domain knowledge and AI expertise. We therefore aim to identify and address challenges related to CCA and explore the opportunities AI offers for data-driven discovery.
Data-driven discovery is enabled by data-intensive research collaborations that go beyond disciplinary boundaries, and include (big) data infrastructures, new sensing technologies and AI algorithms. Recent examples (outside WUR) that attracted media attention include major advances in long-term, complex scientific problems, as for example in protein structure prediction and precipitation prediction. Such solutions, using AI together with domain knowledge, outperform disciplinary solutions in scientific benchmarks and competitions. Another recent example of data-driven discovery is a recent development by BioNTech that partnered with an AI start-up to quickly identify potential high-risk COVID-19 variants: they developed an early warning system that combines structural modelling of spike proteins with AI to detect and monitor high-risk SARS-CoV-2 variants, with almost all COVID-19 variants receiving World Health Organisation designation on average two months prior to official designation.
Over the past decade, data science (DS) and artificial intelligence (AI) in particular have opened new avenues in various application domains, from language translation to robotics and from medical imaging to self-driving cars. Such innovations are based on new algorithms that enable computers to learn patterns from huge data sets and turn them into evidence for further decision-making in new situations. AI is having a major impact on how we do science today. Data-driven discoveries are enabled by data-intensive research collaborations that go beyond disciplinary boundaries, involving (big) data infrastructures, new sensing technologies and AI algorithms.

Climate change adaptation (CCA)
Climate change is already affecting ecosystems, food systems and social and economic systems around the world. Future risks are expected to further exacerbate already vulnerable systems. To date, most climate research, policies and actions have focused on climate change mitigation, i.e. reducing greenhouse gas emissions. However, given the already observed impacts of climate change and the expected increasing risks, any realistic climate strategy also requires an emphasis on climate change adaptation (CCA). Adapting to a changing climate involves taking action to prepare for and adapt to both the current effects of climate change and predicted risks in the future.
Data-driven discoveries are expected to help address the major challenges of our time, and there are several initiatives to achieve this goal, both nationally and internationally. Dere are several examples that demonstrate interest in developing AI for climate change. However, most initiatives originate from the AI community and develop separately from domain knowledge, hindering their full potential for data-driven discoveries. An integrated approach, encompassing domain knowledge with AI expertise, is needed to achieve major breakthroughs. Moreover, while AI has been extensively researched for climate mitigation solutions, there is less experience internationally on how DS and AI methods can contribute to the development of new climate change adaptation solutions.
Wageningen University &Research is uniquely positioned internationally and nationally to fill this research niche. Recent investments in DS/AI give WUR the basis to develop a strong profile combining domain knowledge and AI expertise. We therefore aim to identify and address challenges related to CCA and explore the potential of AI for data-driven discovery. This can both further strengthen WUR's leading position in the world in developing CCA solutions and establish WUR as a leader in impactful AI applications.