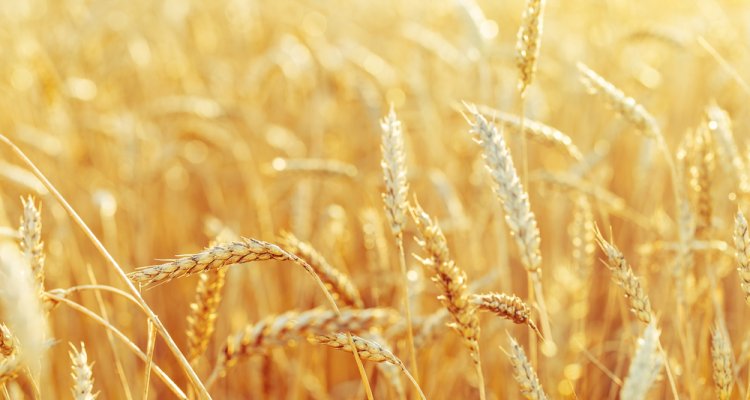
Project
Machine learning for selecting crop varieties as climate adaptation measure
Climate change affects food security worldwide. Local conditions for growing crops change. An actionable strategy for farmers is to plant new varieties that are better suited to these new conditions. This project uses hybrid machine learning for identifying crop varieties that are better adapted to climate conditions at specific locations. We develop methods that combine modelling and statistical approaches to learn from time series data of greenhouse and field observations as well as satellite and drone imagery.
The optimal growing conditions for any crop differ per variety. This is because the varieties of a certain crop differ in their genetic background, which means they will respond differently to local conditions within a growing season. For food security reasons we want to continue producing sufficient yields under climate change. For that we need to identify which existing or new varieties are better suited for new local environments.
Project description
Quantitative tools are essential in the assessment of how varieties will respond to new climates. We combine statistical and machine learning methods for Quantitative Trait Loci analysis and data smoothening (Pérez-Valencia et al., 2022) to identify critical marker genes and essential genotype-by-environment interactions with numerical modelling for quantifying these genotype-by-environment interactions that occur during the growing season. In particular, we use time series data at higher resolutions for this identification. The models are intended to extrapolate how existing and new varieties will perform under new environmental conditions.
Based on fitting to high-resolution time series trial data involving multiple varieties in different environments in Australia across 31 years (Bustos-Korts, 2019), we propose a set of dynamic models of low-complexity that can capture essential environmental limitations to growth. These include local variation in solar radiance and soil water availability, compared to a standard minimalistic logistic model (see Figure 1; Van Voorn et al., to appear). These models are used in a Bayesian framework for the simultaneous fitting of multiple varieties to identify differences between varieties. In turn, this can be used to construct functions that include the interaction effects of multiple marker genes with environmental limitations, to accommodate the extrapolation on how new genotypes will perform in new environmental conditions.
Publications
-
Combining Crop Growth Modeling and Statistical Genetic Modeling to Evaluate Phenotyping Strategies
Frontiers in Plant Science (2019), Volume: 10 - ISSN 1664-462X -
A two-stage approach for the spatio-temporal analysis of high-throughput phenotyping data
Scientific Reports (2022), Volume: 12, Issue: 1 - ISSN 2045-2322