Social networks in pigs based on computer vision
WIAS Magazine - Spring edition 2023
Research Overview
Keywords: welfare, social behaviours, social networks, computer vision, tracking, algorithm, modelling
Background
Social network analysis (SNA) is a powerful tool to quantify inter-individual interactions and identify the social roles of each individual within a group [1]. This method has become a popular approach to study the social dynamics of animal populations and its application has considerably increased in recent years. In livestock, previous social network studies mostly relied on manual observations with a small sampling rate often focusing on one type of social interaction or on proximity. Recent advances in the fields of data science and computer vision offer new opportunities to develop an image-based automatic monitoring tool to automatically detect behaviours at the individual level in groups of animals such as pigs. By giving the pig’s location in each video frame, tracking algorithms give the opportunity to calculate proximity to pen mates. However, multiple options are available to define proximity and decisions need to be made to build social networks. Furthermore, tracking algorithms, by giving information at the frame level, generate a very large amount of data and may require a long computing time. Therefore, down sampling of video frame rates is necessary to study social networks based on tracking algorithms, in order to reduce computing time and to make data accessible in real-time to researchers, breeders, or farmers. However, little is known about the effect of using different proximity definitions and frames rate on the social networks of farm animals.
Approach
In this study, we will use the output of a tracking algorithm (YOLOv3 combined with SORT) from a previous study [2] to detect the location of each individual using bounding boxes (Figure 1). The study will focus on the data collected over three consecutive days, in 21 pens, each containing six pigs. Networks will be constructed with five different definitions of proximity based on distances between the centers of the bounding boxes or the percentage of overlap of the surface of the bounding boxes (Figure 2). The data will be filtered to integrate proximity in the social network only when the two interacting individuals were inactive. We will analyze whether individuals exhibit similar network positions across these social networks based on different proximity criteria. Networks with different sampling rates will be built and we will explore how down sampling, i.e. reducing frame rates, affects the centrality parameters of the individuals.

Figure 1 - Example of a frame with annotated bounding boxes
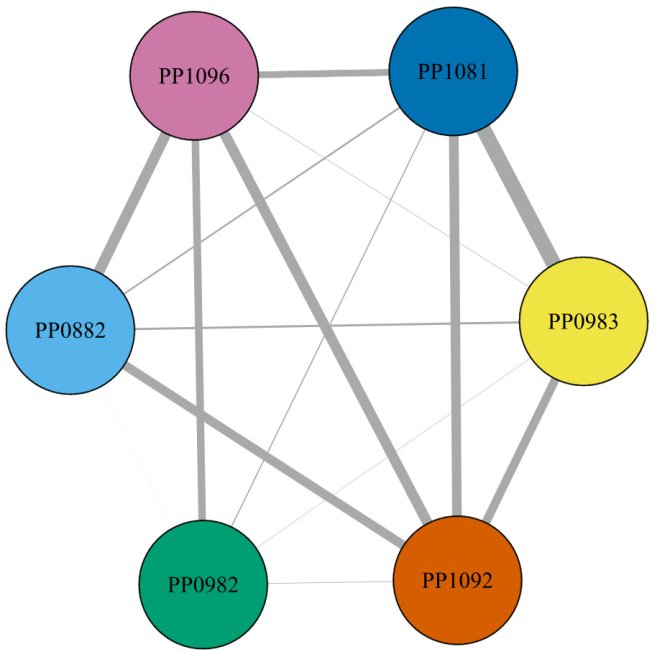
Figure 2 - Example of a proximity social network (Proximity definition: overlap of bounding boxes <20%)
Perspectives
With this study, we want to highlight the variety of choices that are available for researchers to build social networks based on tracking algorithms and how different choices might affect the results and the conclusions drawn. In parallel with this study, within the IMAGEN project, we are collaborating with computer scientist experts from Eindhoven Technical University (Tu/E), to automatically detect the actors and the receivers of social behaviours (with a focus on tail and ear biting). The output of this algorithm will enable us to investigate whether individuals display consistent positions within the network across time (being a victim or a biter) and whether the position is influenced by individual characteristics (for example sex, weight, personality, or genetic background). This approach may open doors to new management or breeding strategies to improve group functioning and prevent harmful behaviours in pigs [3].
References
[1] Makagon, M.; McCowan, B.; Mench, J. How Can Social Network Analysis Contribute to Social Behavior Research in Applied Ethology? Applied Animal Behaviour Science 2012, 138 (3–4), 152–161.
[2] Zande, L. E. van der; Guzhva, O.; Rodenburg, T. B. Individual Detection and Tracking of Group Housed Pigs in Their Home Pen Using Computer Vision. Frontiers in Animal Science 2021, 2, 669312.
[3] Finkemeier, M.-A.; Langbein, J.; Puppe, B. Personality Research in Mammalian Farm Animals: Concepts, Measures, and Relationship to Welfare. Frontiers in Veterinary Science 2018, 5, 131.