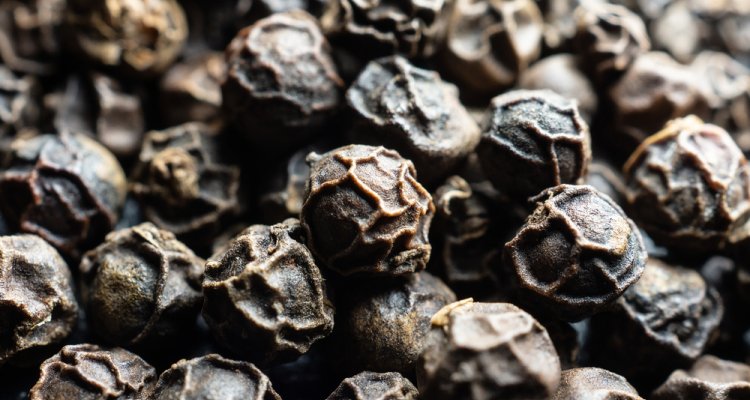
Showcase
Using federated artificial intelligence to overcome data sharing barriers to better detect food fraud
Many companies are generating relevant data for food authentication during their quality control protocols, as proof against food fraud. To do so, they scan the food using sensors for features of authenticity. Subsequently, an AI system models the characteristics of what defines the authenticity, and can help to detect food fraud. The AI model can only be trained with large amounts of data, so called big data. Big data is usually created by combining several smaller sets which can be owned by different companies. However, barriers to data sharing can hinder the creation of a sufficiently large data set. So, how can we train the AI system without first sharing data.
WUR scientists Jasper Engel, a statistician at Wageningen Plant Research-biometrics (WPR), and Martin Alewijn, a food chemist at Wageningen Food Safety Research (WFSR), are exploring how federated learning can help to detect food fraud. For this purpose, they are developing novel algorithms to apply AI technology for food fraud detection without sharing sensitive data, while helping all parties in the value chain, including consumers.
Quality control up to date
The use case in this research is about black pepper from different companies. The goal is to use additional information from fellow companies to improve their own model for quality control. However, these data are seen as the companies property and as sensitive information. Besides that, the challenge of the AI model is to determine clear boundaries of what is, in this case, black pepper and what is not. The aim of the AI model is to determine when a batch is altered with something that looks like that food, intentionally or by accident. The computer learns the (natural) specific food composition more broadly with a larger dataset, becoming better in detecting deviations. This is important as the composition of the black pepper, changes in time due to several factors such as growing conditions, ripening stage at harvest, storage and processing conditions, or cultivars. The natural variance in black pepper is relatively wide in the context of international trade, where the pepper is grown in several countries and supplied by millions of small-scale farmers. Pepper batches change in time and the database must be kept up to date. The larger the dataset, the more accurate the model can stay. During a previous research project, the improvement of a sensor was executed. However, the willingness of the companies to share data was limited. Therefore, the idea of using federated learning to improve the model was born.
Create a win-win situation
The project consists of a proof of principle using real data from several companies. The data consist of quality scans by either the same sensor type and brand or two different sensors types combined, all anonymized. It allows companies to jointly develop an AI model for food authentication within disclosing their own data which are seen as company-sensitive information. This development creates a win-win situation. All companies involved beneficiate of the additional data to better detect food authenticity and learn from each other anonymously, having no negative impact on their competitiveness on the market.
And it works!
Jasper: “During this project, we have demonstrated that with our novel federated learning algorithm for food fraud, we can get the same results as if you would have had access to all the data. Those results are more accurate than if each company would use only their small dataset to tackle the issue.” By using federated learning, the data is packaged so that it becomes non-sensitive, so in this example, we can make food safer and detect more fraud. But what is federated learning exactly?
It is like the EU, we are working together but separately
Federated learning is a sub-field of machine learning. Each company can scan their pepper samples for authenticity, using their local AI model. At the same time, an extract of the data, without the sensitive information, is shared and used to improve the global model, from which the local models learn. Martin: “Federated learning is already used in other sectors such as medical sciences and banking, where files are anonymously used. They cannot share personal data, but they do want to learn from others sensitive information in the value chain."
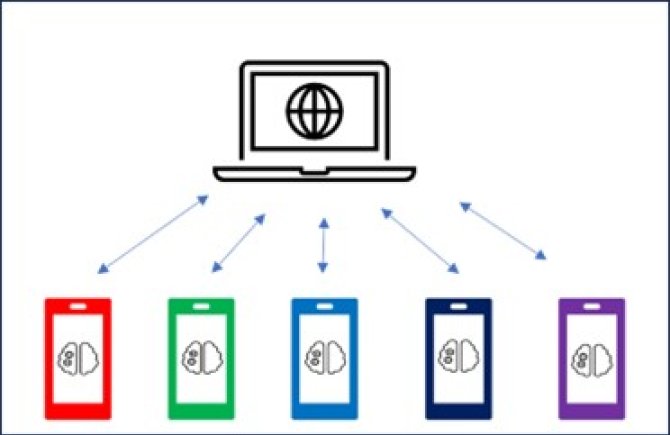
What is next?
There is still more work to be done before implementing the model. Implementing is essential to reflect real use. Furthermore, the road to implementation will bring its load of practical questions such as how to organize the data. Jasper hopes to be able to help people using the model by answering those practical questions, whereas Martin hopes that WFSR can be a trusted organisation by the other stakeholders for the coordination of the data base and be the link between all parties for the coordination of the model.
A bigger project is needed to test the federated learning principle in everyday life. A decision model could also be coupled to the federated model. This way, all analysis done could be used by the model and get more out of the gathered data in terms of information and fraud detection. This is how artificial intelligence is at the service of food authenticity.”