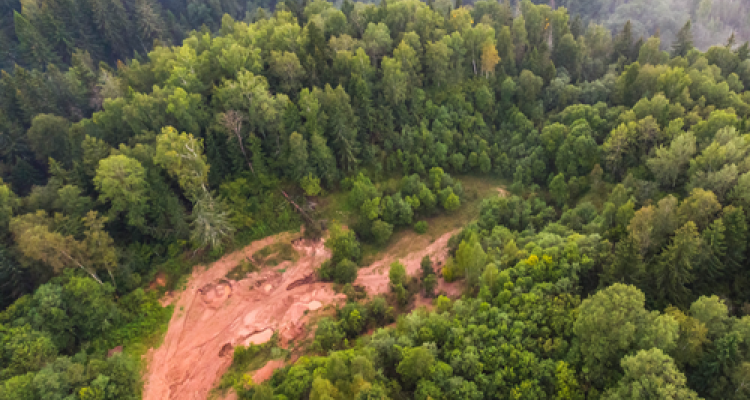
Colloquium
Analyzing forest disturbance/recovery cycles in Peruvian forest ecosystems using the AVOCADO algorithm
By Marieke Buuts
Abstract
Forests play a vital role in providing crucial ecosystem services, but are facing degradation due to human activities and increased natural disturbances. To tackle this problem, monitoring efforts like REDD+ are focused on reduction of carbon emission from deforestation and degradation. These monitoring efforts require a robust approach for disturbance and regrowth detection. The recently developed AVOCADO algorithm offers a continuous and semi-automated approach for disturbance and regrowth detection, while capturing seasonal and gradual changes. As the performance of the algorithm varies among different areas due to variations in phenological patterns of vegetation, season, or deforestation drivers, this study aims to assess the performance of the AVOCADO algorithm in different types of tropical forest ecozones in the Peruvian Amazon. These ecozones encompassed a tropical lowland forest, a tropical montane forest, and a tropical wetland forest ecozone. The focus is on detecting human-induced deforestation events and subsequent regrowth, evaluating ecozone-specific adaptations of the AVOCADO algorithm for improved detection of forest disturbance and recovery in these ecozones. The study explores eight vegetation indices and user-defined algorithm parameter settings.
Each ecozone type had a selected study site of approximately 10 km2. For these study sites, Landsat-derived vegetation index time series imagery ranging from 1990 until 2021 were acquired. For each index, a phenological reference curve was established from an undisturbed, natural reference forest area within the study site. The study found that the best-performing index for change detection varied among ecozone types. The selection of optimal performing parameter settings was influenced by the quality of Landsat imagery available in each ecozone type. The findings indicate that suitable index selection and parameter fine-tuning area crucial for obtaining highly accurate detection results. However, due to variations in phenological patterns at larger scales, the algorithm’s application to larger areas may be limited.
The study achieved high overall accuracies (ranging from 76.4% to 96.9%) for both disturbance and regrowth change detection across all three ecozone types, demonstrating the algorithm’s potential in monitoring initiatives like REDD+.