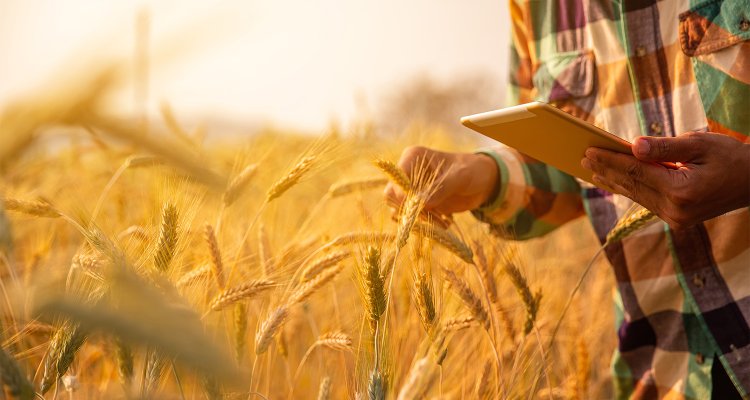
Project
Federated data for food system predictions
Federated data and learning offer transformative potential for the food system, facilitating data sharing, accurate forecasting, and data privacy. This project pioneers their application in agrifood, establishing a foundation for future work. Despite budget constraints, it aims to create a framework and proof-of-concept for food system parameter prediction.
Federated data and learning are considered promising technologies that hold the potential to bring substantial benefits to the transformation of the current food system. These include increased incentives and capacities for data sharing, enhanced food system parameter forecasting accuracy, and improved preservation of data privacy.
Federated data and learning have traditionally found applications in high-tech and healthcare industries, while their utilisation in the agrifood domain has been comparatively limited. This project aims to pioneer the application of such technology in the agrifood sector, establishing a foundation for its implementation in this field.
Due to budget constraints in 2023, our priority will be establishing a strong foundation for a potential follow-up project in 2024. This will involve creating a framework to guide the implementation of a federated-data-based food system prediction and a proof of concept. The detailed deliverables in 2023 will be:
A framework will be established to provide clear guidance for the effective implementation of federated data and learning methodologies within the agrifood domain. This framework aims to serve as a foundational pillar to facilitate the transformation of the food system through advanced technological integration and collaborative data-driven approaches.
First step of ontology development within WUR domain to facilitate federated data & learning. Ontology development within the WUR domain is of high importance in enabling the integration of federated data and learning methodologies. This important step sets the groundwork for bringing together different types of data. This, in turn, forms the basis for a strong system that combines data and learning, ready to change how we gather and use knowledge together.
A proof-of-concept will be created involving building a federated learning model using available data sources to predict a parameter related to the food system