Project
EUCARPIA Biometrics
We were glad to welcome you to the XVIth Meeting of the EUCARPIA Section Biometrics in Plant Breeding. This meeting hosted scientific presentations on the development and application of quantitative methods and strategies in plant breeding. A major topic was the use of genome-wide marker data to predict phenotypic performance, coined “genomic prediction”, in plants and crops with invited speakers from human and animal genetics. Furthermore, the meeting provided great opportunities to talk to leading experts and to meet researchers and breeders of other companies and organizations.
9-11 September, Wageningen, The Netherlands

Download Group Photo
Documents

Abstracts of invited speakers
Peter Visscher (Centre for Neurogenetics & Statistical Genomics, University of Queensland, Australia) - Genomics and big data in human populations: combining genetics and epigenetics to predict phenotypes

Driven by advances in genome technologies, the last 8 years have witnessed a revolution in our understanding of complex trait variation in human populations. Results from genome-wide association studies and whole-genome exome studies have shown that the mutational target in the genome for most traits appears to be very large, such that many genes are involved in explaining genetic variation. Genetic architecture, the joint distribution of the effect size and frequency of variants that segregate in the population, is becoming clearer and differs between traits. We will show new results from disparate complex traits including height, schizophrenia and gene methylation, to illustrate polygenicity and the power of experimental sample size. In addition, we will show emerging results that epigenetic information can be used to make predictions of complex traits and that gene methylation can be a predictor of past environmental exposures.
Neil Hausmann (Dupont Pioneer, United States) - Future Breeding Systems: view from DuPont Pioneer

John Hickey (University of Edinburgh, United Kingdom) - Sequence to Phenotype: Allocation of Resources

Background. Genomic selection is increasingly valued within the plant breeding community. To implement genomic selection large investments are needed in genomic data (markers and or sequence) and phenotypic data on which to train prediction equations. Choices about distributing these resources affect the return on investment.
Results. A simulation was conducted which evaluated the long term benefit of three alternative breeding program designs: (i) a classical plant breeding program design; (ii) a minor modification to the classical design in which genomic prediction was used to increase the accuracy of preliminary yield trials; and (iii) a complete reorganization of the breeding program into a population improvement component driven by genomic selection and a product development component that was similar to i.
Conclusions. The different breeding program designs gave different returns on investment. Complete reorganization of plant breeding programs into population improvement components driven by genomic selection and product development components was promising but its benefit was affected by costs.
Emma Huang (CSIRO Computational Informatics and the Food Futures National Research Flagship, Australia) - Meta-alleles in multiparental populations
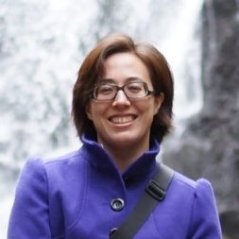
Multiparental populations have become increasingly popular in plant breeding due to their high genotypic and phenotypic diversity. In particular, MAGIC populations, which mix the genomes of multiple founders through several generations of recombination, offer relatively high resolution and power for investigation of many traits simultaneously.
Typically, models for QTL mapping in such populations follow two approaches, testing association either with the observed marker genotypes or the unobserved founder genotypes. If there is a single causal variant and it is genotyped, or is in strong linkage disequilibrium with a genotyped marker, then the first, simpler model is the most powerful possible test. The second, full model, allows each founder of the population to have a different effect, thereby allowing for multiple causal variants. However, it may be over-specified since it is unlikely that all founders have different effects.
Models intermediate in complexity that elucidate the number of distinct functional alleles should better represent the true genetic architecture of the trait, particularly in testing for interactions, where the number of effects in the full model can quickly outnumber the size of the population.
We consider here three approaches to collapsing founder alleles into ‘meta-alleles’. The first, based on clustering haplotypes in sliding windows based on genomic similarity was proposed by Leroux et al. (2014). This data-driven approach was shown to have highest impact in a scenario with a huge number of medium/small-size families. We propose two alternate approaches with biological interpretations of the meta-alleles which may be more appropriate for MAGIC populations. One determines the set of distinct isoforms of each protein encoded by the founders of the population. These “protein alleles” are used to cluster the founders. The other clusters founders based on time to the most recent common ancestor.
We compare all three approaches to the simple and full models through application to a four-parent wheat and 19-parent Arabidopsis MAGIC population. Further, we perform simulations based on the Arabidopsis population to quantify the gains achievable through use of these methods.
Jens Riis-Jacobsen (CIMMYT) - Accelerate genetic gain by taking advantage of additional data sources and integrated data analysis – case studies from maize and wheat breeding at CIMMYT
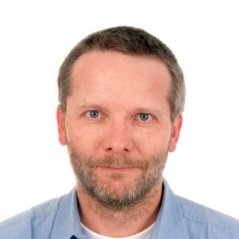
Background: Exploitation of plant genetic resources is dependent on germplasm related data being transformed into useful information that supports decision making and enhances genetic gain. Traditional data sources in the breeding work are genealogy and phenotypic data, but in recent years additional sources such as genotypic data, environmental data, and different sensor data have become available at a low cost. Nevertheless so far, only the largest breeding companies have managed to take advantage of the new sources of data in integrated informatics and analytics platforms, and the majority of organizations involved in plant breeding are struggling with how to harness this potential. Taking the maize and wheat breeding programs at CIMMYT as the point of departure this paper analyses how a small to medium sized breeding institution can take advantage of new data sources, what benefits they may obtain, and what some of the challenges involved are.
Findings:
· Genealogy and phenotypic data remains the foundation data for crop genetic improvement, and with available tools it is possible to setup the core elements of a future integrated breeding information system
· Genotyping, climate, and remote sensing data can make valuable contributions in a plant breeding program as has been demonstrated with ad hoc studies, but tools that facilitate mainstreaming of this in plant breeding programs are not generally available
· While the informatics and biometric challenges in an integrated breeding platform are being addressed, plant breeding institutions will still be faced with challenges related to establishing a multidisciplinary team as well as change management capabilities that can implement the solutions
: New data sources and new analytical capabilities like high throughput phenotyping can accelerate genetic gain, and plant breeding programs that incorporate these may benefit from added productivity. Informatics and biometric solutions are increasingly available, which will lower the barriers for using integrated approaches. However, the full potential will only be realized when breeding activities and investments are reorganized and smaller breeding programs may also struggle to access the broader set of competencies required.
Alison Smith (University of Wollongong, Australia) - Experimental designs for expensive multi-phase traits
The importance of sound experimental designs for plant breeding trials cannot be underestimated. They are crucial to ensure valid inference and accurate prediction of genetic effects, whether they be effects for traditional or genomic selection or the identification of QTL. Many key traits involve multi-phase experiments, where grain samples are taken from a field experiment (Phase I) then processed further in one or more laboratory experiments (Phase II and higher). Typically the laboratory phases are costly relative to the field phase and this necessitates a limit on the total number of samples that can be tested. Historically this has been achieved by sacrificing field replication and testing a single composite sample for each variety, obtained by combining grain from all field replicates. Typically no replication or randomisation is employed in the laboratory phases. In this talk we describe the approach of Smith et. al. (2014) in which replication is achieved in all phases of the experiment. In terms of field replication, some varieties are tested as composite samples and some as individual replicate samples. Replication in the laboratory is achieved by splitting a relatively small number of field samples into sub-samples for separate processing. Model-based design techniques are used to obtain efficient designs for the laboratory phases, conditional upon the field design. Unlike the historical approach, this method allows the application of an efficient statistical analysis to the resultant data so that accurate predictions of genetic effects may be obtained.
The approach will be illustrated using an Australian wheat quality project that involved a series of field trials and subsequent measurement of a range of flour, dough and end-product traits.
A major challenge with this project was to develop experimental designs and protocols that were not only statistically valid, but also satisfied strict budgetary constraints and were pragmatic, in the sense of complying with standard laboratory practice. We show how all of these issues were successfully addressed using the approach of Smith et al. (2014).
Andres Gordillo (KWS) - Genomic selection strategies and validation in hybrid maize and rye
Hans Peter Piepho (Biostatistics Unit, Universität Hohenheim) - The generation of efficient row-column designs for field trials

Luc Janss (Aarhus University) - Genomic analysis in tetraploid potato using genotyping-by-sequencing
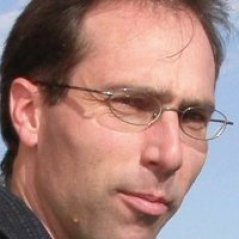
Dave Marshall (The James Hutton Institute) - The data challenges from the application of high throughput technologies in plant breeding and genetics
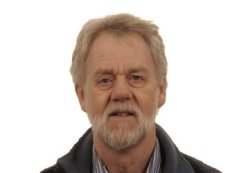
Marco van Schriek (Keygene ) - Exploitation of digital phenotype markers for prediction of brassica napus field seed yield
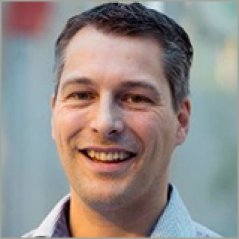
Controlled phenotyping platforms or conventional pot trials are often used to compare plant performance under water stress and non-stressed conditions. Studies which compare stress reactions of plants under these controlled conditions with performance in the field are rare. Correlations between pot experiments and field trials are essential in order to identify and exploit morphological or physiological selection criteria for practical breeding approaches. To this end a selection of diverse winter oilseed rape cultivars known to show variable stress responses in the field were screened. All cultivars we grown in irrigated and non-irrigated field trials at multiple locations in Germany. The same cultivars were also grown under water-stressed and non-stressed conditions in two controlled experiments. Firstly a container experiment where the experiment was performed over a complete growing season so that seed yield could be measured. For the second experiment the cultivars were tested by digital phenotyping using the PhenoFab system. I will present correlations between early digital phenotypes and field yield-relevant parameters observed in this study.