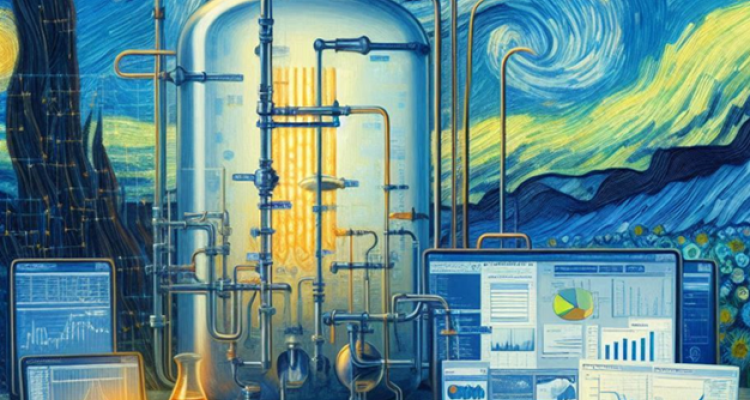
Project
BioIndustry 4.0
The biomanufacturing industry has the prospect of embracing the latest industrial revolution, also known as Industry 4.0, which holds the promise for innovative solutions to achieve sustainability objectives. In order to undertake this challenge, a physical-to-digital-to-physical information loop should be meticulously developed. A strategy to achieve this is by implementing Digital Twinning. Digital Twins (DT) which are digital replicas of a process. In order to boost its development, it is essential to standardize knowledge, data extraction from the physical object and to develop computational approaches to aid in the design and real-time optimization of the physical object, the bioprocess. However, DT in biomanufacturing is falling behind the other sectors of industry in terms of its implementation. Therefore, the objective of this project is to pave the way towards digitalized and bio-intelligent operation systems in Bio-manufacturing.
Background information
The sector of industrial biotechnology is currently in a maturation phase, at the same instant the industrial landscape is constantly evolving to meet the growing demands and maintain competitiveness. Hence, biotechnology has to adapt in order to remain relevant and viable. In this context, the new industrial era is shifting from traditional manufacturing to digitalized manufacturing. This transformation harnesses real-time process data to control processes autonomously and precisely at their optimal state. Such control is critical in reducing or maintaining production costs, enhancing yields of product on substrates, and ensuring consistent product quality (Gerzon et al., 2022).
Digital Twins and data-driven process monitoring, operation, and optimization, represent the main two areas of developments in manufacturing, resulting from the advent of digitalization and Industry 4.0 movement (A. Udugama et al., 2021). These advancements play a catalytic role in enhancing the efficiency and viability of bioprocesses. In summary, the development of Digital Twins progresses from foundational steady-state models to dynamic and validated simulations, concluding in real-time integrated systems that enhance operational efficiency and decision-making in biomanufacturing. The evolution from Digital Models to Digital Shadows and ultimately to Digital Twins reflects increasing complexity and capability, driven by advancements in real-time data acquisition, predictive modelling, and optimization algorithms.
However, the path towards a digitalized bioeconomy hinges not only on technological integration but also on advancing the performance of cell factories. Digitalization alone, regardless of its extent, will not suffice without the enhanced performance of these cell factories. Thus, advancements in cell factory capabilities are essential to realize the full potential of a digitalized bioeconomy.
Project description
The main aim of this project is to design and operate an upstream bioprocess, encompassing the strain development, while creating several computational tools that will facilitate real-time monitoring and control of the bioprocess. These tools will facilitate real-time optimization of the bioprocess while helping identifying potential improvements of the microbial platforms on a genetic level. Process, constraint-based and kinetic modelling will be developed and utilized to simulate the bioreactor and the strain of interest. While, machine learning approaches will be employed as well, to further improve the prediction of outcomes over time, and to generalize the mechanistic understanding to larger datasets. Consequently, a Digital Twin should integrate both the mechanistic and the statistical components to enable both explanatory and predictive capacities. Throughout this process, strain engineering will also implemented in order to improve the characteristics of the bioprocess.
In summary, the five steps approach will be taken in to account toward implementing a comprehensive Digital Twin.The process begins with the creation of a Steady State Model (Step 1). It advances to a Dynamic Model (Step 2) represented by differential equations indicating changes over time. The next stage involves validating the model (Step 3). Step 4 introduces the Digital Shadow, which continuously updates the model parameters. Finally, Step 5 achieves Model-Based Control where control actions are derived based on real-time data and predictions.