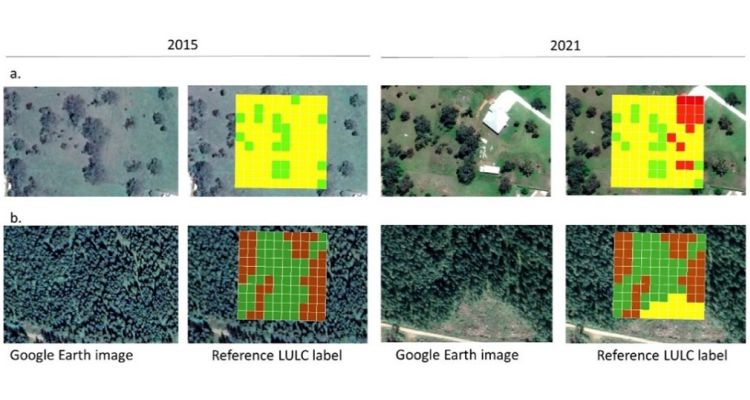
Thesis subject
MSc thesis topic: Unlocking the Value of Existing Reference Datasets: A Remote Sensing Approach
Remote sensing based land monitoring is an important part of implementing and assessing various policies targeting climate change mitigation and sustainable future. Remote sensing based land monitoring frequently uses reference datasets to train, calibrate and validate maps depicting different aspects of the earth's surface, e.g., forests, land use, land cover, and soil types. High-quality reference datasets are necessary for accurate results, and they can be used to train machine learning algorithms and also to validate the results.
The reference datasets are commonly created using a manual labelling process that is a time-consuming task. Thanks to open science initiatives, many open reference datasets are available, however, they may be outdated as they are not maintained regularly. Therefore, their re-use has been limited, although significant efforts are required to collect reference data (Pengra et al. 2020; Tarko et al. 2021). Considering the knowledge these existing reference datasets hold, it is important to improve their quality and reuse for remote sensing applications.
Different remote sensing methods can be used to detect outdated reference sample points. The possible methods include the dense time series based algorithms such as the BFAST (Breaks for Additive and Seasonal Trend)(Verbesselt et al. 2010) that is used to flag sample sites with possible change (Tsendbazar et al. 2021), assessing the inconsistency in reference dataset based on remote sensing reflectance (Fonte et al. 2020) and convolutional neural network that is to assess the quality of reference data (Can et al. 2019).
To better exploit existing reference datasets, this thesis will assess the use of different remote sensing methods for updating reference datasets.
Overall aim
- To improve the re-usability of reference datasets using remote sensing techniques.
Research question
- Which remote sensing methods are suitable to be used to identify and update reference labels with change?
- To what extent remote sensing methods can be used to update reference datasets?
Literature
- Can, R., Kocaman, S., & Gokceoglu, C. (2019). A convolutional neural network architecture for auto-detection of landslide photographs to assess citizen science and volunteered geographic information data quality. ISPRS International Journal of Geo-Information, 8, 300
- Fonte, C.C., Patriarca, J., Jesus, I., & Duarte, D. (2020). Automatic Extraction and Filtering of OpenStreetMap Data to Generate Training Datasets for Land Use Land Cover Classification. In, Remote Sensing
- Gong, P., Liu, H., Zhang, M., Li, C., Wang, J., Huang, H., Clinton, N., Ji, L., Li, W., Bai, Y., Chen, B., Xu, B., Zhu, Z., Yuan, C., Ping Suen, H., Guo, J., Xu, N., Li, W., Zhao, Y., Yang, J., Yu, C., Wang, X., Fu, H., Yu, L., Dronova, I., Hui, F., Cheng, X., Shi, X., Xiao, F., Liu, Q., & Song, L. (2019). Stable classification with limited sample: transferring a 30-m resolution sample set collected in 2015 to mapping 10-m resolution global land cover in 2017. Science Bulletin, 64, 370-373
- Pengra, B.W., Stehman, S.V., Horton, J.A., Dockter, D.J., Schroeder, T.A., Yang, Z., Cohen, W.B., Healey, S.P., & Loveland, T.R. (2020). Quality control and assessment of interpreter consistency of annual land cover reference data in an operational national monitoring program. Remote Sensing of Environment, 238, 111261
- Tarko, A., Tsendbazar, N.-E., De Bruin, S., & Bregt, A.K. (2021). Producing consistent visually interpreted land cover reference data: Learning from feedback. International Journal of Digital Earth, 14, 52-70
- Tsendbazar, N., Herold, M., Li, L., Tarko, A., de Bruin, S., Masiliunas, D., Lesiv, M., Fritz, S., Buchhorn, M., Smets, B., Van De Kerchove, R., & Duerauer, M. (2021). Towards operational validation of annual global land cover maps. Remote Sensing of Environment, 266, 112686
- Verbesselt, J., Hyndman, R., Newnham, G., & Culvenor, D. (2010). Detecting trend and seasonal changes in satellite image time series. Remote Sensing of Environment, 114, 106-115
Expected reading list before starting the thesis research
- The same as above
Requirements
- Advanced remote sensing
- Geoscripting
- Interests to work in Google Earth Engine
Theme(s): Integrated Land Monitoring