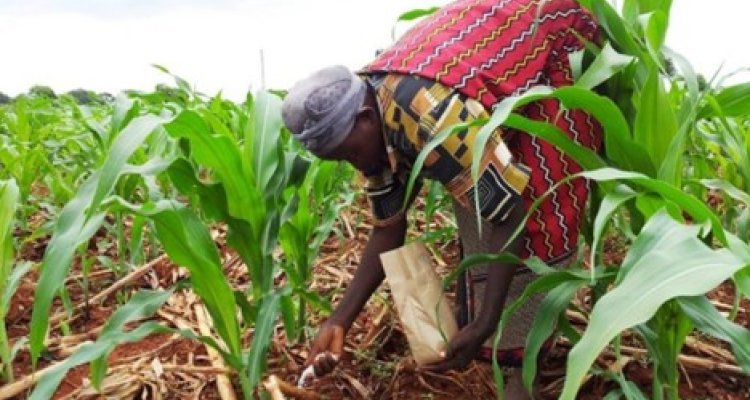
Student information
MSc thesis topic: Incorporating uncertainty in spatial decision making
Spatial data play a key role in various decision-making contexts, including spatial planning, ecosystem management, and land degradation mitigation. Decision-makers encompass local or national governments, companies, organizations, but also individuals who must make spatially informed choices. For example, smallholder farmers in Africa might confront decisions concerning land management, such as determining the necessity and profitability of fertilization. Moreover, many decision-makers exhibit risk aversion, prioritizing certainty or lower-risk alternatives over higher-risk options, even if the latter offer a potentially higher ‘expected’ return.
Spatial data are rarely, if ever, free from errors, which can significantly impact spatial decision-making processes. Thus, a fundamental question emerges: how can decision-making be optimized in scenarios characterized by imperfect and uncertain spatial information? This thesis research will focus on optimizing fertilizer recommendations for farmers, by analysing how uncertainty regarding soil nutrient concentrations influences decisions regarding fertilization recommendations.
Objectives and Research questions
- The overall objective is to develop a statistical methodology that supports spatial decision making under uncertainty and to test the methodology in a real-world fertilizer recommendation case study.
Possible research questions are:
- In what way can the optimization criterion used in decision-making processes be generalized so that it also works in cases where input variables are uncertain?
- Which is a suitable computational algorithm to optimize the criterion?
- What results are obtained when the methodology is applied to a real-world case study of land fertilization and how do these results compare to a case where uncertainty is ignored?
- Can the methodology also accommodate risk-aversive decision makers and what methods are suitable to have decision makers quantify their risk-aversion?
Requirements
- Having passed the ‘Spatial Modelling and Statistics’ course is desired because much of the required methodology has been covered in that course.
Literature and information
- Readers of the ‘Spatial Modelling and Statistics’ course.
- General text books, such as https://mitpress.mit.edu/9780262029254/decision-making-under-uncertainty/
- In-depth scientific articles, such as https://doi.org/10.1016/j.spasta.2022.100619
Theme(s): Modelling & visualisation