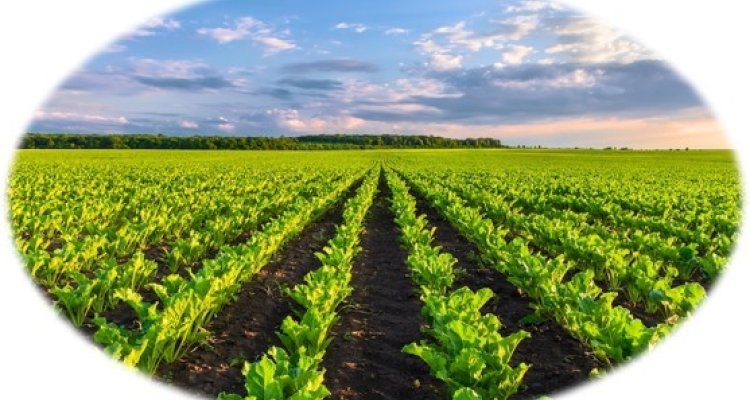
Colloquium
Reconstruction of NDVI time series based on SAR data using a deep learning method
By Yongiin Wang
Abstract
The demand for agricultural production output is continuously increasing in the world of today, while climate change, disasters, and other issues threaten agricultural production and food security, placing higher demands on agricultural management. As a means of remote monitoring, remote sensing is widely used in agricultural monitoring due to its low cost and high efficiency, playing an important role in assisting agricultural management. The Normalized Difference Vegetation Index (NDVI) is a vegetation index calculated by the red and near-infrared bands of optical remote sensing images. It is closely related to crop growth and productivity characteristics, making it valuable in agricultural monitoring. However, optical remote sensing is highly sensitive to weather conditions and prone to cloud cover, leading to frequent data gaps in NDVI time series generated based on it. Since synthetic aperture radar (SAR) is independent of weather conditions, and SAR data has been proven to be related to NDVI, reconstructing the NDVI time series from SAR data is a potential solution. This study is based on the U-net model, which combines different Sentinel-1 SAR data and auxiliary data to train nine models. These models are used to reconstruct the NDVI time series of several crops in the Flevopolder region of the Netherlands in 2022, and are validated on the temporal scale. The modified Cropmarkers algorithm developed by Wageningen Environmental Research (WENR) is applied to the reconstructed NDVI time series to detect crop emergence, closure, transition, and harvest stages, thus validating the performance of the trained models in practical agricultural applications. The method used in this study achieves the best overall results for full canopy crops, with the corresponding R2 values obtained for sugar beet, consumption potato, silage maize, and winter wheat being 0.92, 0.87, 0.67, and 0.82, respectively. Worse results were obtained for half canopy crops, with the R2 values for tulip and onion being 0.56 and 0.67, respectively. This is probably due to the low proportion of half canopy crop parcels in the training dataset resulting in insufficient training. On grassland, the changes in NDVI data caused by mowing could not be predicted well. For full canopy crops, the reconstructed NDVI time series detected the four crop penological stages relatively well. The average deviation for crop emergence and closure ranged from 5 to 10 days, and for transition it was between 10 to 15 days. For harvest it varied from around ten to over twenty days depending on the crop type, while for half canopy crops the results were worse. Among the nine models, Model M51, which used multi-temporal backscatter data, crop identification maps, and year and week information as input layers, achieved the best overall performance, whereas adding 12-day interferometric coherence data did not improve model performance. Compared to the results of other literatures utilizing traditional machine learning algorithms, the method used in this study significantly improved the quality of the reconstructed NDVI time series, demonstrating the applicability of the U-net model over a larger spatial extent, and providing a solution for supplementing optical NDVI time series.